
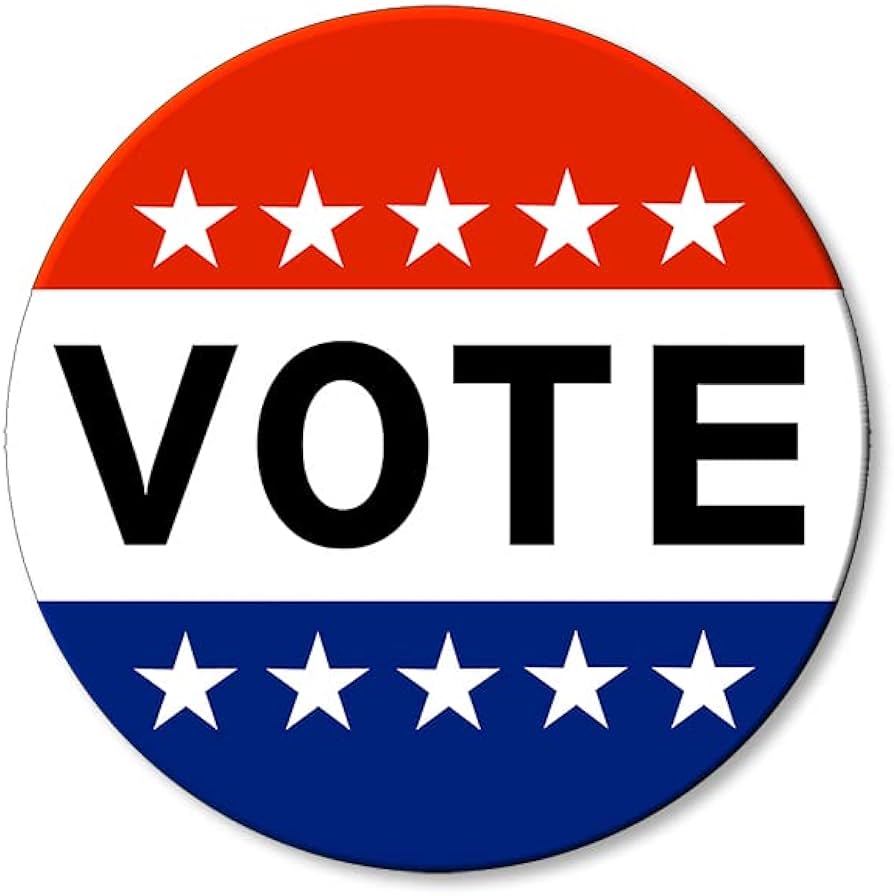
List of sources quoted in this list of "push back:
- A group called “Republicans against Trump” which seems to just be this twitter account with a buymeacoffee link which posted “Republicans could choose this man to represent them, instead they preferred a corrupt racist buffoon with no morals. The party deserves a humiliating defeat in 2020.” In May 2020, making me suspect they’re not actually Republican or possibly a group at all.
- “A Republican accountability group called American Bridge 21st Century” which is described on Wikipedia as “a liberal American Super PAC that supports Democratic candidates and opposes Republican candidates.”.
- The American Federation of Labor and Congress of Industrial Organizations
- A “popular liberal commentator”
- A Democratic Representative
- The White House political director
So if you were hoping for actual consequences from his base or even just someone new and noteworthy criticizing him, this is not the article for you. I’m glad the Trade Unions are going to spread the word though, that will be a good thing.
For anyone else also interested, I went and had a look at the links Dessalines kindly provided.
The source on the graphs says “Sources: Daniel Cox, Survey Center on American Life; Gallup Poll Social Series; FT analysis of General Social Surveys of Korea, Germany & US and the British Election Study. US data is respondent’s stated ideology. Other countries show support for liberal and conservative parties All figures are adjusted for time trend in the overall population.” Where FT is financial times.
It’s not clear how the words “liberal” and “conservative” were chosen, whether they’re intended to mean “socially progressive” and “socially traditional” or have other connotations bound with the political parties too, and whether the original data chose those descriptions or if they’re FT’s inference as being “close enough” for an American audience.
Unfortunately the FT data site is refusing to let me look at them without “legitimate interest” advertising cookies so I can’t tell you much more or if there’s any detail on methodology.